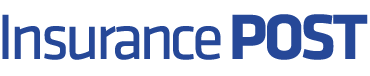
The evolution of risk modelling in insurance
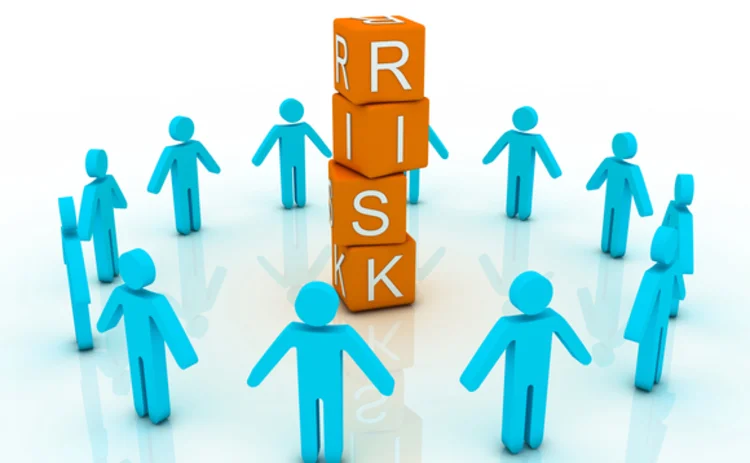
Need to know
- Solvency II forced insurers and reinsurers to consider what risk models they were using
- Cloud computing is allowing insurers to run risk models quicker using more variables
- Insurers cautioned about using AI and machine learning just because of the hype
- Insurers and brokers recruiting more data scientists and retooling existing actuarial staff to take advantage of risk modelling oppotunities
Regulation might have helped to speed up the evolution of risk modelling but technology advances, especially cloud computing, are now taking this development to the next level. Edward Murray explores the benefits this is bringing and how insurance companies are upskilling to take full advantage.
It might not be possible to predict the future, but the exponential increase in data availability and computing power means risk modellers can generate quicker and more accurate forecasts than ever before.
Brokers and insurers are hungry for the information these predictive models generate as it feeds into virtually every aspect of their businesses.
Regulators have also accelerated the evolution of risk modelling by introducing mandatory requirements for insurers to better understand and quantify the risks they underwrite.
So just how quickly is the risk modelling market moving? How is changing technology impacting the way risk modellers work? What new skill sets are risk modellers using, and into what new areas is risk modelling expanding?
Market on the move
Coping with significant and regular changes to regulation has been one of the defining challenges of the insurance market over recent years. One major piece of legislation to hit the statute books was Solvency II, which came into effect on 1 January 2016.
A part of the new rules put demands on insurers to quantify their liabilities more consistently and accurately that stipulated minimum reserving and capital adequacy levels.
Insurers have always sought to create the most accurate and sophisticated view of their exposures and potential liabilities, but the demands enshrined in Solvency II put an additional focus on these risk modelling activities and accelerated the existing direction of travel.
Adam Podlaha, head of impact forecasting at Aon Benfield, agrees: “Solvency II forced insurers and reinsurers to examine what models they were using, why they were using them, and to understand what it meant to them as a business. It provided an extra motivation for companies that were moving in this way anyway.”
The legislation also demanded that insurers and reinsurers got better and faster at risk modelling in the ensuing years, stipulating shorter timeframes in which reports had to be filed.
Joel Fox, risk consulting and software, global life product and solution leader at Willis Towers Watson, explains: “In 2016 some companies went live with the minimum possible, but because the reporting deadlines were due to reduce there was pressure to improve what was in place. In the first year companies had eight weeks to submit their quantitative reporting templates. It then fell to seven, six and five.”
He adds: “It is post-Solvency II that companies which had been compliant realised they needed to make changes to meet the five-week deadline.”
Now that companies have met the regulatory requirements there is a growing appetite to focus more on the commercial benefits of risk modelling. These have never been ignored, but they have had to play second fiddle to achieving compliance.
As companies refresh and evolve their risk modelling capabilities, they are also beginning to think about how they can use improving technology to their advantage and use risk modelling data throughout their entire business.
Changing IT
For hundreds of years the insurance market has set premium rates on its policies by calculating the likely claims costs they will generate. Historically, that work has been done by insurers looking backwards at previous claims and then mapping that claims experience forward to fit the evolving risk environment.
Experience and previous risk performance remain at the heart of risk modelling, but improved IT capabilities have enabled the insurance industry to start thinking on a grander scale. These capabilities include better compatibility between risk modelling platforms, increased computing power, and lower costs.
The latter two benefits are largely a result of the opportunities created by cloud computing, although there is still some way to go before the industry realises the potential benefits on offer.
Fox explains: “To date I do not think people have really changed the nature of their calculations as a result of the cloud. It has more been about doing it faster and cheaper. Looking ahead, when companies take a breath, they will realise what they can now do on a massive scale at a sensible cost and start changing the sorts of calculations they do – more intricate, more regular, more variations and so on.”
One development that is necessitating more computing power to run risk models is the inclusion of more numerous and detailed images.
Brendon Sussams, catastrophe risk manager at Allianz UK, says: “With advancements in graphics processing units and availability of images such as aerial and satellite, the models we are using are becoming more sophisticated and therefore, larger. This makes them more difficult to handle and, to ensure timely analysis, we are always looking for the most efficient platform to host them. Some of the move towards cloud is being driven out of necessity with the next generation of natural catastrophe models requiring cloud deployment due to their size and complexity.”
The exponential increase in computing power is also enabling risk modellers to refine their workings and to test them more effectively for accuracy.
Mike Palmer, head of analytics and research at Hiscox Re, says: “Sensitivity testing the models has become more feasible – you can change one or other of the input parameters and see what happens and you can do that on a vast scale. It has been possible to do this before, but it has taken a long time to complete these tasks.”
He adds: “In the past, there might have been a queue and things went through one at a time, in simple terms. In a cloud deployment it is possible to do many more things in parallel and so it improves your ability to generate faster throughput and to complete increasingly complex analyses.”
As risk modellers get better at identifying the relationship between individual risk factors and subsequent claims costs, the more accurately insurers will be able to price their policies and make appropriate reserving decisions.
The speed at which models can now be run, is also enabling insurers to use the insights they generate almost in real time and for a wider variety of purposes.
Nirav Shah, head of pricing and analytics at Tokio Marine Kiln, says that risk modelling results are no longer being used on a monthly or quarterly basis. He comments: “With the advent of cloud computing and distributed computing you can produce results much quicker and essentially on the fly. If these results are available, then we should be using them.”
Emphasising the importance of speed in generating these results he adds: “Techniques such as marginal pricing or portfolio optimisation need you to produce results on an ongoing basis. If results are to be accurate and relevant for when they are needed, you have to be able to run your risk models in a live timeframe.”
In addition to increases in speed and accuracy, which cloud computing is helping to achieve, today’s digital is making it possible to access wider data sets.
Shah says: “It is becoming easier to integrate with more data sources and services and so the industry is not purely reliant on the same old sources that it has always used. There is more lateral thinking going on. What about census data, government data, data from social media, and from sensor devices?”
However, Shah is the first to urge caution and he believes there is a need to resist seeing new data sources and emerging IT advances, such as artificial intelligence and machine learning, as silver bullets.
He explains: “Across the industry, AI is developing very quickly but I would like to urge some caution. With machine learning and AI, as an industry we are not being very mature with how we deploy these capabilities. We are caught up in the hype that it is cool and fashionable, but I do not think we have defined our use cases very well or how we can monetise them.”
The challenge for risk modellers is to remain focused on the problems they are trying to solve and to ensure that IT is deployed in a controlled way so that it creates genuine value. Given the almost endless possibilities created by AI, data availability and computing power, this is no easy task.
Upskilling for the future
As technology becomes increasingly central to risk modelling, there is a need for brokers and insurers to reappraise the skillsets of those they have working on these models.
Insurance and actuarial expertise will always be central to designing risk models, but there is a growing need to recruit additional specialists.
Fox says: “We have brought more data scientists on board and we are also training our actuaries in some of the new techniques and methodologies.
Palmer adds his company has done the same thing and is now using data scientists to try and get a new perspective on the data available and the ways in which it could add value to the organisation.
Tokio Marine Kiln is sending its actuarial analysts back to university to complete a master’s degree in data science, while broker Marsh is another company looking to upskill its analysts and build a deeper in-house understanding in advancing IT techniques.
Raj Lakhani, senior vice-president at the broking firm, comments: “We have set up this workstream where we upskill our current analytics team members to enable more proficiency in machine learning. That is ongoing, and we have set it up so that each member of the group, over the next year, has to upskill themselves as well as find a business case in which they identify a problem that needs solved and come up with a solution for it.”
As brokers and insurers recruit employees with new skills and improve the capabilities of their existing team members, they are also uncovering unintended, but very useful benefits.
Certainly, this is the case at Tokio Marine Kiln and Shah explains: “We have a project on the go where we are looking at our data ingestion and how we make it available to the business given where it comes from. This is purely a process problem – not a risk modelling problem – but we are getting involved. Our data scientist is now looking at our data flows and helping our data management team optimise the flow of information through our business until they get to the end stage whether that is reporting, analytics, or modelling.”
Risk modellers would not normally get involved in such process-driven projects, but Shah says new knowledge within his team is allowing the risk modelling department to increase its relevance and importance to the business as a whole.
New areas of influence
In addition to the benefits of having data scientists embedded within an organisation, improved risk modelling is also helping the insurance sector create better outcomes in a number of areas.
One is in emerging lines of business where the liabilities are not fully understood and there is only a short claims history to fall back on.
Cyber insurance is a classic example, and brokers and insurers are using today’s more sophisticated risk modelling techniques to try and get a handle on their exposures and liabilities.
Palmer says the company is also using its risk modelling expertise to pave the way into new underwriting opportunities.
He explains: “Hiscox is very interested in exploring the potential for US flood insurance as the market begins to free up. It is a very difficult peril to model and understand because it varies on such short geographical scales. If there is a flood in Texas, for example, a house on one side of the street might be flooded while properties on the other side are unaffected. Dealing with the complexities of that very short spatial scale has recently become possible in a sensible timeframe.”
The skills developed by risk modellers are also delivering benefits in other areas as Sussams says: “Geospatial modelling brings operational efficiency gains from routing our surveyors to distribution assessment. Scenario modelling is often employed to complement existing models in part to sense check their output, for example, flood accumulation modelling, or for emerging risk types, or where an area of need has been identified.”
Risk modelling has always been a core function within the insurance industry. However, improving technology and more accessible data sets mean its importance is growing.
Where brokers, insurers and reinsurers get their risk modelling strategy correct and make the most out of the potential opportunities available, they will significantly improve the products and services they offer and steal a march on competitors.
Only users who have a paid subscription or are part of a corporate subscription are able to print or copy content.
To access these options, along with all other subscription benefits, please contact info@postonline.co.uk or view our subscription options here: http://subscriptions.postonline.co.uk/subscribe
You are currently unable to print this content. Please contact info@postonline.co.uk to find out more.
You are currently unable to copy this content. Please contact info@postonline.co.uk to find out more.
Copyright Infopro Digital Limited. All rights reserved.
You may share this content using our article tools. Printing this content is for the sole use of the Authorised User (named subscriber), as outlined in our terms and conditions - https://www.infopro-insight.com/terms-conditions/insight-subscriptions/
If you would like to purchase additional rights please email info@postonline.co.uk
Copyright Infopro Digital Limited. All rights reserved.
You may share this content using our article tools. Copying this content is for the sole use of the Authorised User (named subscriber), as outlined in our terms and conditions - https://www.infopro-insight.com/terms-conditions/insight-subscriptions/
If you would like to purchase additional rights please email info@postonline.co.uk