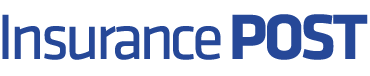
This article was paid for by a contributing third party.
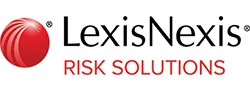
Blog: Model behaviour for insurance risk prediction - destroying the myth of the magic funnel
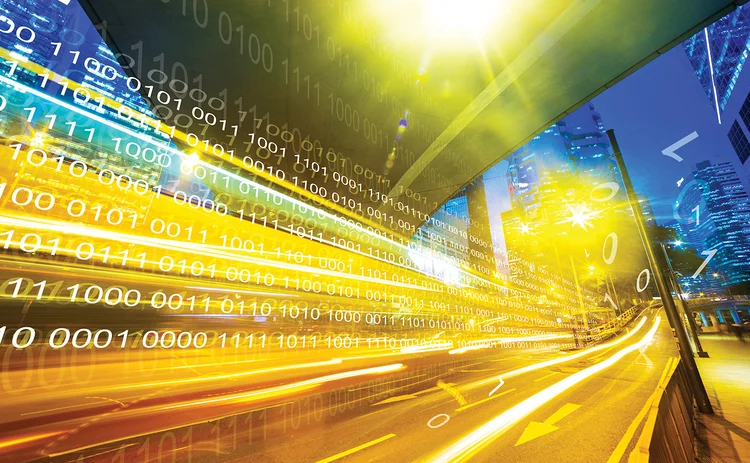
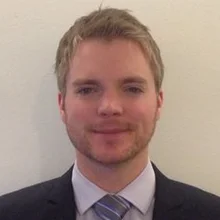
The Financial Conduct Authority announced in September that it is planning to launch a market study into how general insurance firms price home and motor insurance, following a super complaint by the Citizen Advice Bureau to the Competition and Markets Authority.
With the UK insurance sector coming under further regulatory pressure, the time would seem ripe to re-evaluate the use of data to inform pricing strategies and perhaps take a back to basics approach to help ensure the right foundations are in place for risk modelling. This way insurance providers can build the most accurate possible picture of risk – for both new and existing customers.
For insurers and brokers looking to broaden either their use of data or re-evaluate their current data models, understanding the difference between good and bad information and how to model that data is fundamental.
More data points are likely to become available as the industry evolves – consider contributed policy history and past claims data, smart home data, connected car data. For insurance providers to maximise and leverage the opportunities these new data sources bring through data enrichment at point of quote and renewal, they need to go back to their initial data sources.
This can start with refreshing the initial data model, as the original rating plan used may have been written one or two years previously. By refreshing the data, it will identify behaviours used in rating the risk, how it’s changed and how it should be adapted for the current market.
Without this crucial first step, adding in new data could be duplicating effort and capturing behaviours that existing models could actually acquire. Insurance providers could find that information from an existing data source and a new one is very similar – almost like double counting – and this has a negative impact on the modelling.
It is also worth viewing new data sources as possible replacements for existing data sets rather than an add-on to the current data used. Insurance providers need to look at the incremental benefits a new data source will bring.
If the data ticks the right boxes in terms of consistency, completeness, the desired market coverage, for example, the process of modelling can start. We believe this should always be from a retro-analysis perspective.
Once the base model has been refreshed, care needs to be taken over the structure of the analysis and the order in which the data sources are compared. Taking data source one and adding data source two will provide a different outcome compared to taking data source two and adding data source one.
The key is to look at the data through the same lens. Therefore, when statistical modellers are testing a data model set, a ‘combined model’ approach could be the solution.
Using public data from a mix of sources, such as credit reference agencies and other data providers, can help an insurance provider understand its best credit model. Then, adding other data sources like policy history, or named driver in motor insurance, may help identify any additional uplift and take those learnings to price more accurately and help rule out risk of cancellations for brokers.
It’s generally advisable to avoid modelling on missing data. The structure and enrichment of the data depends on the level of filtration required and more importantly, the outcome being sought. For example, a broker could be using data to predict cancellations, whereas an insurer could be looking to predict claim losses.
When it comes to filtration, modellers want to ensure they have a full picture of the exposure they are modelling on, for example, if a policy has not run for a full year that data may be omitted or adjusted.
There is no simple formula where all data is put into a magic funnel that draws out the desired outcome. The process of data enrichment, filtering and structuring relies heavily on the initial data sources and how they are modelled.
As the industry continues to rise to the challenge of pricing in a highly competitive market, maximising the opportunities of its data models to create more intelligible insights and a strategic advantage over competitors is vital.
Sponsored
Copyright Infopro Digital Limited. All rights reserved.
You may share this content using our article tools. Printing this content is for the sole use of the Authorised User (named subscriber), as outlined in our terms and conditions - https://www.infopro-insight.com/terms-conditions/insight-subscriptions/
If you would like to purchase additional rights please email info@postonline.co.uk
Copyright Infopro Digital Limited. All rights reserved.
You may share this content using our article tools. Copying this content is for the sole use of the Authorised User (named subscriber), as outlined in our terms and conditions - https://www.infopro-insight.com/terms-conditions/insight-subscriptions/
If you would like to purchase additional rights please email info@postonline.co.uk